Exploring the Role of SOM Interneurons in Cortical Response Modulation
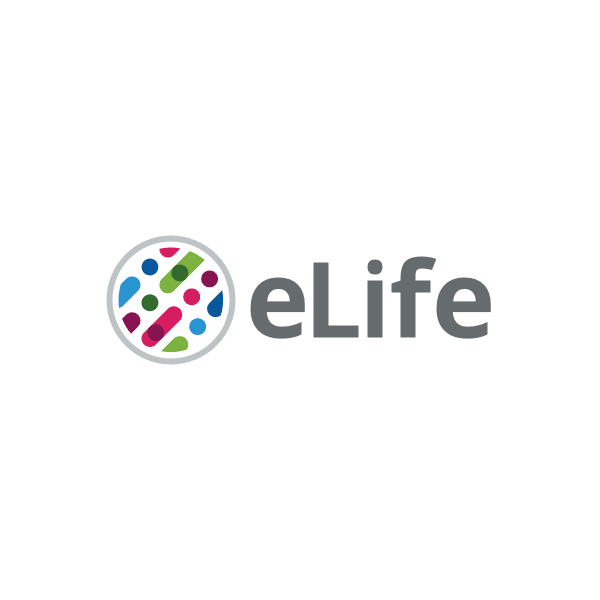
Recent research has provided compelling in vivo evidence underscoring the pivotal function of somatostatin (SOM) interneurons in modulating cortical responses. Studies conducted by Urban-Ciecko and Barth (2016) and Yavorska and Wehr (2016) demonstrate this critical role. However, deciphering the intricate wiring between excitatory neurons and inhibitory neurons, as explored by Tremblay et al. (2016), Pfeffer et al. (2013), Jiang et al. (2015), and Campagnola et al. (2022), poses significant challenges for researchers aiming to understand the specific mechanisms by which SOM neurons influence cortical activity.
To better gauge the impact of SOM inhibition on excitatory neuron (E) responses, researchers typically consider two distinct inhibitory circuit pathways. These are: a direct inhibitory pathway where SOM neurons inhibit E neurons, and a disinhibitory pathway, where SOM neurons inhibit parvalbumin (PV) neurons, which in turn affects E neuron activity.
Experimental evidence has highlighted contrasting effects of SOM neurons on E neuron activity. In one notable study, increased activity of SOM neurons led to decreased excitatory neuron firing rates in the layer 2/3 of the mouse visual cortex, as reported by Adesnik et al. (2012) and further explored in 2017. Conversely, research conducted by Canto-Bustos et al. (2022) and Wang and Yang (2018) observed that a reduction in SOM neuron activity resulted in increased E neuron activity in the piriform cortex. This paradoxical response has prompted researchers to delve deeper into the physiological and circuit properties that dictate the E PV SOM circuit's behavior, particularly focusing on why heightened SOM activity can either suppress or enhance E neuron responses.
Addressing this question necessitates an understanding of the entire recurrent connectivity present within the E PV SOM neuron circuit, rather than solely examining the SOM E and SOM PV E interactions. To facilitate this, researchers established a recurrent network model delineating the firing rates of E, PV, and SOM neurons. This approach mirrors methodologies employed in previous studies focusing on the E PV SOM cortical circuit, as referenced by Litwin-Kumar et al. (2016), Kuchibhotla et al. (2017), and more recent works from Mahrach et al. (2020) and Veit et al. (2023).
In the proposed model, the fundamental distinction between PV and SOM neurons is that PV neurons have the capacity to inhibit one another, whereas SOM neurons do not exhibit this self-inhibition. Additionally, while PV neurons process external sensory inputs, SOM neurons respond to modulatory inputs. The researchers then sought to investigate how modulating SOM neuron activity (via I S m o d) would influence E neuron activity ( r E m o d). Various forms of modulation were explored, including the suppression of vasoactive intestinal peptide (VIP) inhibition on SOM neurons, activation of pyramidal cells that preferentially project to SOM neurons, and direct cholinergic modulation of SOM neurons.
The dynamics of the model revealed a nonlinear response, with each neuron population exhibiting a complex nonlinear transfer function. This behavior aligns with numerous experimental findings reported by Priebe and Ferster (2008) and Romero-Sosa et al. (2021). To elucidate the parameters that influence the direction of E rate changes, researchers utilized a linearization approach around a defined dynamical state of the model. This allowed for the definition of a cellular gain (b X) associated with each neuron class, with X representing the neuron types {E, P, S}. Additionally, it involved a comprehensive examination of the entire circuits dynamics, as highlighted by Garcia del Molino et al. (2017) and Litwin-Kumar et al. (2016).
By thoroughly evaluating the influence of SOM on E neurons through all potential synaptic pathways, the researchers articulated that changes in SOM activity can affect E rates via various connections. These include monosynaptic pathways, disynaptic pathways, and even trisynaptic pathways. Fortunately, the researchers were able to simplify this complex relationship into two key network motifs that ultimately determine the direction of E rate changes. These motifs embody both the disinhibitory elements of the network and the inhibitory components.
The researchers determined that the overall connectivity and input parameters could result in either a decrease or increase in E rates, depending on the specific conditions of the network. For example, certain configurations align with neuronal recordings in layers 2 and 3 of the visual cortex, while alternative configurations correspond with increased E rates as observed in layer 4 neurons of the somatosensory cortex. This analysis provided a valuable framework for discussing the mechanistic reasons behind this disparity. In essence, variations in the response of E rates to SOM modulation can arise from different factors, including direct inhibitory effects or disinhibitory effects, variations in self-inhibition levels of PV neurons, or disparities in their firing rates.
In conclusion, while the E PV SOM recurrent circuit showcases a complex array of polysynaptic pathways, the interaction between inhibitory and disinhibitory pathways fundamentally dictates the modulatory influence of SOM neurons on E neuron activity. This comprehensive understanding opens avenues for future research, particularly in exploring how these pathways impact network stability and the stimulus-response dynamics of excitatory neurons.