In-Depth Analysis of RNA-Sequencing Data for S. aureus CC8 Clade
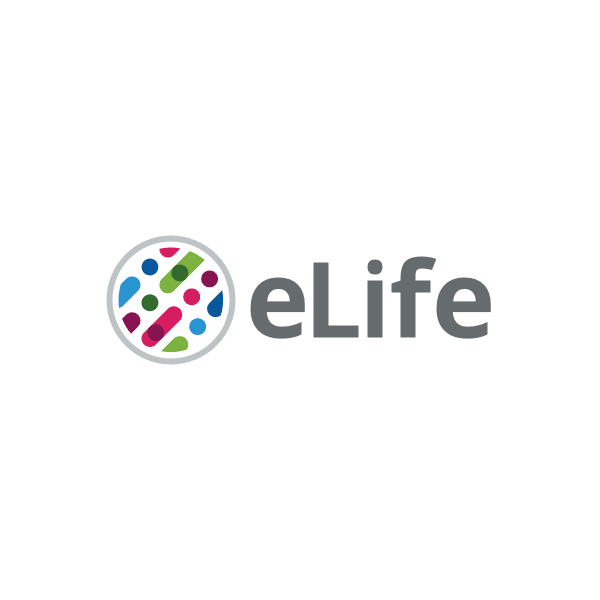
The intricate world of RNA sequencing and its implications in understanding Staphylococcus aureus, specifically the CC8 clade, has been further unraveled through a recent study utilizing the pymodulon package. As outlined by Sastry et al. (2021), the research team meticulously gathered all available RNA sequencing data corresponding to both non-USA300 and USA300 S. aureus strains. This data was subjected to a comprehensive Quality Control and Quality Assurance (QC/QA) pipeline, ensuring that the metadata was manually curated and the sequences aligned accurately to the reference genome of TCH1516 (NC_010079, NC_012417, and NC_010063).
The analysis involved transforming the combined RNA-sequencing data into log-TPM (transcripts per million) values, followed by normalization against a specific reference condition (SRX3760886 and SRX3760891). This method illustrates a notable departure from other Independent Component Analysis (ICA) models that typically normalize data to project-specific reference conditions, a process that often leads to the loss of strain-specific information. This is critical since many BioProjects only encompass data from individual isolates, such as NCTC8325, TCH1516, and LAC, limiting the potential insights into strain-specific behaviors.
To further dissect this, the research team executed ICA following a pre-established protocol to generate iModulons specifically for the CC8 clade of S. aureus, as previously demonstrated by Sastry et al. (2019). The first step involved collecting a comprehensive set of RNA-sequencing data and corresponding metadata for the S. aureus strains identified within the CC8 clade. Most of these sequences were derived from well-known strains like TCH1516, FPR3757, LAC, Newman, and NCTC8325, although some were labeled generically as USA300 while still belonging to the CC8 lineage.
The fastq files obtained from these samples were processed using TrimGalore (v0.6.5) to trim sequences, and subsequently aligned to the reference TCH1516 genome employing bowtie2 (v1.2.3) (Krueger, 2015; Langmead and Salzberg, 2012). Gene-specific read counts were computed using HTSeqCount (v2.0.1) through strict intersection criteria, followed by normalization to TPM and log-transformation into log-TPM format.
Prior to leveraging the data, rigorous assessments of read quality and alignment were conducted using FastQC and MultiQC (v 1.11) (Andrews, 2010; Ewels et al., 2016). Samples failing to meet quality benchmarks such as 'per base sequence quality', 'per sequence quality score', 'per base n content', or 'adapter content' were excluded from further analysis. Furthermore, any samples with fewer than 500,000 reads aligned to the reference genome were discarded. The final selection process also involved removing samples lacking replicates or those exhibiting Pearson correlation coefficients below 0.9.
In total, the study narrowed down 670 RNA-sequencing samples, collecting extensive metadata that included growth conditions, genetic alterations, and experimental associations. The log-TPM values were centered around the reference condition of S. aureus TCH1516 grown in RPMI+10% LB, allowing the ICA to effectively capture strain-specific regulatory modifications. For instance, the ICA methodology adeptly identified the activity of the Fur transcription factor, representing it as a linear combination of a Fur iModulon and a second iModulon that highlighted differences between USA300 and non-USA300 strains.
The research team utilized FastICA to compute the M and A matrices from the centered log-TPM data, revealing the structure of iModulons and their respective activities (Pedregosa, 2011; Koldovsk et al., 2006). To determine the optimal model, a careful computation of stable components was necessary. Given that FastICA is non-deterministic, it produces slightly varied component weightings and activity levels across iterations, sometimes yielding spurious components present in only a subset of runs. To filter out stable components, the team conducted ICA 100 times with a randomized seed.
Components that emerged consistently across iterations were identified through clustering with DBSCAN. Careful attention was also given to the number of components specified for data decomposition. An insufficient number could amalgamate signals from multiple transcription factors into single components, while excessive decomposition might generate numerous unstable, single-gene iModulons likely capturing noise. To ascertain the optimal number of components, the heuristic method OptICA was employed, testing various input component counts from 10 to 340 and suggesting an optimal number that minimized single-gene iModulons while maximizing robust components (McConn et al., 2021). Ultimately, the final model comprised 270 input components, with 148 deemed robust.
In each identified component, genes were classified as part of an iModulon if their weightings deviated from a Gaussian distribution, as determined by DAgostinos test. Each iModulon underwent comparison with genomic features (such as regulons, phages, mobile cassettes, etc.), establishing associations based on significant overlaps between the two groups (hypergeometric test; adjusted p-value <0.05, precision 0.5, and coverage 0.2). Additionally, manual curation was performed for other iModulons linked to distinct features, such as designating iModulons with translation-associated genes as Translation iModulon. The final stage involved a detailed parsing of output iModulon activities to pinpoint those exhibiting the most substantial strain-specific variations.