Deciphering the Role of SOM Interneurons in Modulating Cortical Responses
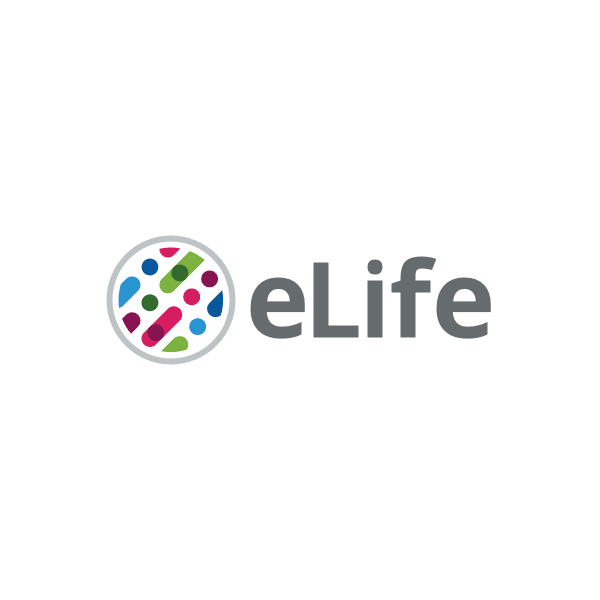
In the intricate world of neuroscience, recent research has unveiled compelling in vivo evidence highlighting the pivotal role of somatostatin (SOM) interneurons in modulating cortical responses. Studies conducted by Urban-Ciecko and Barth (2016) and Yavorska and Wehr (2016) have established the significance of these interneurons. However, the understanding of the specific mechanisms through which SOM neurons exert their influence is complicated by the complex interconnections between excitatory and inhibitory neurons, as noted in the works of Tremblay et al. (2016), Pfeffer et al. (2013), Jiang et al. (2015), and Campagnola et al. (2022). This intricate wiring poses challenges in thoroughly deciphering the functional dynamics of SOM neurons.
Researchers often distinguish between two primary inhibitory circuit pathways when analyzing the impact of SOM inhibition on excitatory neurons (E): the direct inhibitory pathway from SOM to E, and the disinhibitory pathway that proceeds through parvalbumin (PV) neurons before reaching E neurons.
Investigations have yielded seemingly contradictory findings regarding the effects of SOM neuron activity on E neurons. In one notable study, researchers observed that increased activity in SOM neurons directly inhibited E neurons, as demonstrated in experiments conducted on the layer 2/3 of the mouse visual cortex (Adesnik et al., 2012; Adesnik, 2017). Conversely, a reduction in SOM neuron activity led to heightened activity among E neurons in the piriform cortex, as shown in studies by Canto-Bustos et al. (2022) and others (Wang and Yang, 2018). Conversely, other studies suggest that alterations in E neuron activity resulting from SOM perturbations are largely attributed to disinhibitory pathways. For instance, silencing layer 4 SOM neurons within the mouse somatosensory cortex resulted in diminished activity of E neurons (Xu et al., 2013). This dichotomy in the response of E neurons to SOM activity raises critical questions concerning the physiological and circuit characteristics that dictate these outcomes.
Addressing these questions necessitates a comprehensive analysis of the recurrent connectivity within the E-PV-SOM circuit, rather than focusing solely on the individual pathways. To explore this complex interplay, researchers established a recurrent network model that simulates the firing rates of E, PV, and SOM neurons (Figure 1A; see Methods). This approach is consistent with similar studies investigating the dynamics of the E-PV-SOM cortical circuit (Litwin-Kumar et al., 2016; Kuchibhotla et al., 2017; Garcia del Molino et al., 2017; Mahrach et al., 2020; Veit et al., 2023; Palmigiano et al., 2023; Waitzmann et al., 2024; Kumar et al., 2023). In the model, it is crucial to differentiate between the behaviors of PV and SOM neurons: PV neurons possess self-inhibitory properties, while SOM neurons do not. Furthermore, PV neurons respond to external sensory input, whereas SOM neurons are influenced by modulatory signals.
The research team utilized this model to investigate how the modulation of SOM neuron activity, denoted as ISmod, relates to changes in E neuron activity, represented as rEmod. Various forms of modulation have been identified, including the suppression of vasoactive intestinal-peptide (VIP) inhibition on SOM neurons (Pi et al., 2013), activation of pyramidal cells projecting to SOM neurons (Adesnik et al., 2012), and direct cholinergic modulation of SOM neurons (Kuchibhotla et al., 2017; Urban-Ciecko and Barth, 2016).
Figure 1 illustrates the tradeoff between two inhibitory motifs in the E-PV-SOM cortical circuit. (A) A schematic of the complete E-PV-SOM network model, where either positive or negative modulation is applied to SOM neurons. (B) Displays the transfer function and population gain for neuron populations within the network. (C) Depicts the relationship between SOM input modulation ISmod and changes in E neuron rates rEmod. (D) Highlights scenarios where positive SOM modulation results in decreased or increased E neuron rates, depending on the configurations of connections within the network.
The findings indicate that the model response is nonlinear, with each population of neurons exhibiting a nonlinear transfer function (Figure 1B, top). To assess how various circuit parameters influence the sign of changes in E neuron rates, researchers applied a well-established concept of linearization. By linearizing around a specific dynamical state of the model, the researchers were able to define cellular gain bX for neuron populations (Figure 1B, bottom). The network-level linearization encompasses the entire circuit, leading to the formulation of the equation: rEmod = LE, S ISmod. Here, LE, S represents the transfer coefficient correlating SOM and E neuron modulations.
Essentially, LE, S is influenced by the synaptic weight matrix, with each element defined by the coupling between neuron types. The complexity of the parameter space, consisting of twelve variables including synaptic couplings and cellular gains, adds a layer of intricacy to analyzing modulations. The study presents a framework to navigate this complexity effectively.
To elucidate the effect of SOM modulation on E neuron rates, researchers expressed the impact based on all possible synaptic pathways. Conceptually, the influence of SOM modulation on E rates can be interpreted as an infinite sum of synaptic pathways of increasing order (Figure 1C, top). Consequently, changes in SOM rates affect E neuron rates through monosynaptic pathways, disynaptic pathways involving PV neurons, and so forth. Fortunately, the summation can be simplified, revealing that only two network motifs primarily determine the sign of changes in E rates (Figure 1C, bottom). These motifs encapsulate both the disinhibitory and inhibitory components of the network, with the overall behavior contingent on the connection strengths between neurons.
The results indicate that under specific conditions, SOM modulation can lead to a decrease in E rates, corroborating findings from neuronal recordings in layers 2 and 3 of the mouse visual cortex (Adesnik et al., 2012; Adesnik, 2017). Conversely, alternative parameters can yield an increase in E rates, consistent with observations from layer 4 neurons in the somatosensory cortex (Xu et al., 2013). This analysis reveals the intricate relationship between synaptic pathways and their influence on E rate changes, suggesting that discrepancies in E neuron responses could stem from various circuit or cellular factors.
In conclusion, the comprehensive analysis of the E-PV-SOM recurrent circuit highlights the complex interplay of polysynaptic pathways. The identified tradeoff between inhibitory and disinhibitory pathways is critical in understanding the modulatory effects of SOM neurons on E neuron activity. The ongoing investigations will delve deeper into how these pathways influence network stability and the stimulus-response gain of E neurons.