Revolutionary Brain-to-Speech Interface Achieves Near-Real-Time Communication for Paralyzed Individuals
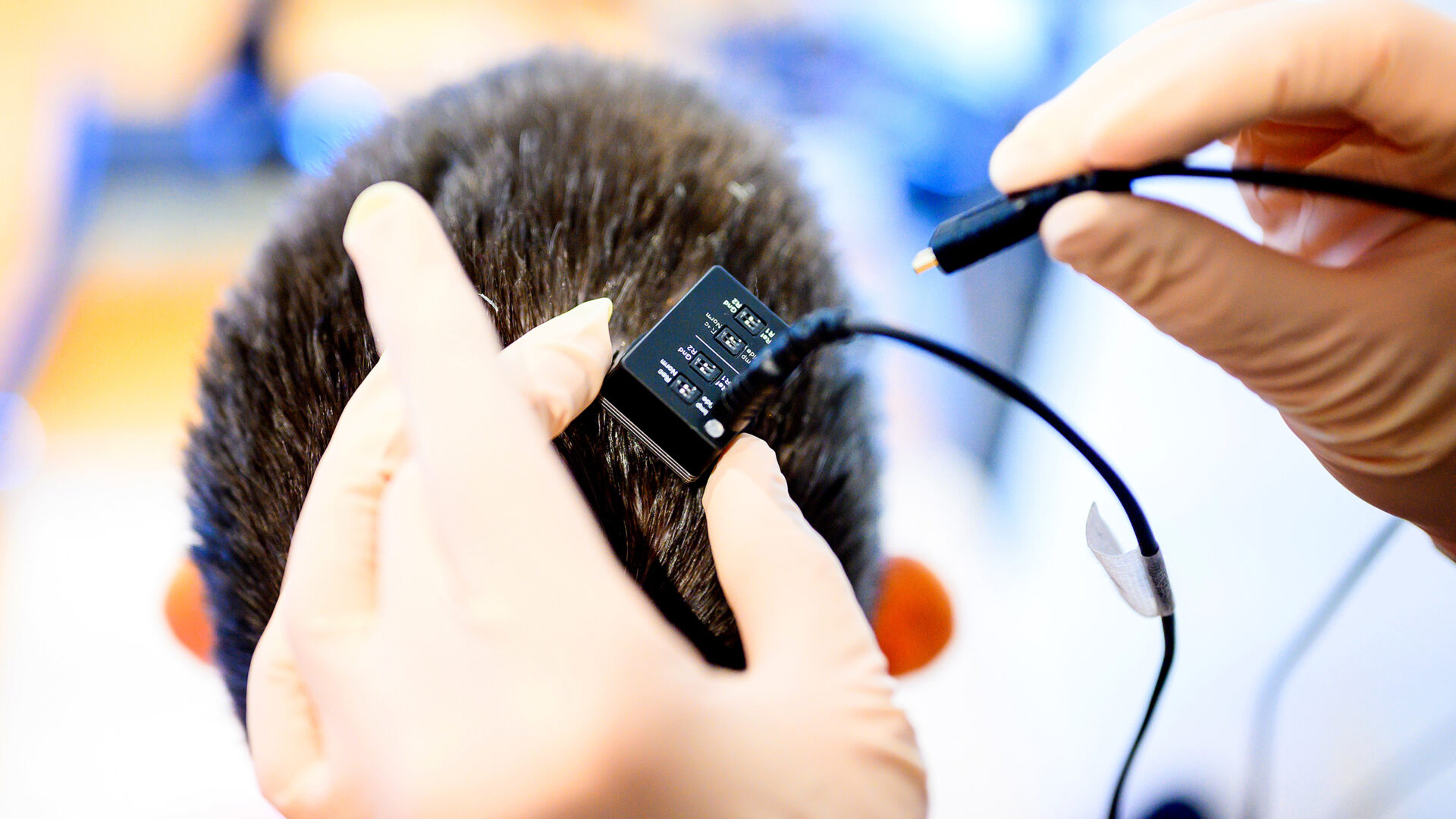
For years, brain-to-speech interfaces have held tremendous promise in assisting individuals with paralysis to communicate effectively. However, these systems have struggled with significant latency, which has hindered their practicality and usability. A dedicated team of researchers from the University of California, Berkeley, and the University of California, San Francisco, have made remarkable advancements in this field by developing a new system that offers near-real-time speech synthesis.
The primary goal of this groundbreaking research was to create a more naturalistic speech experience using a brain implant in conjunction with a voice synthesizer. Historically, attempts to harness this technology faced considerable challenges, particularly concerning latency issues that could leave users waiting for as long as eight seconds for a decoded signal to produce an audible sentence. This excessive delay necessitated the development of innovative techniques aimed at accelerating the process, thereby significantly reducing the wait time between a user's attempt to speak and the hardware's output of synthesized voice.
The researchers developed a sophisticated implant designed to sample data from the speech sensorimotor cortex of the brain. This area is crucial as it governs the mechanical functions involved in speech, including the movements of the face, vocal cords, and other bodily components that facilitate vocalization. The implant captures intricate brain signals using an electrode array that is surgically embedded into the brain. Once the data is collected, it is transmitted to an AI model that decodes these signals and translates them into intelligible audio output. Cheol Jun Cho, a Ph.D. student at UC Berkeley, explains, We are essentially intercepting signals where the thought is translated into articulation and in the middle of that motor control. So what were decoding is after a thought has happened, after weve decided what to say, after weve decided what words to use, and how to move our vocal-tract muscles.
To train the AI model effectively, researchers utilized a participant named Ann, who has experienced paralysis following a stroke, rendering her unable to communicate verbally. During the training process, Ann was instructed to look at prompts and attempt to vocalize various phrases. Remarkably, even though she could not produce sounds, relevant areas of her brain remained active during these attempts. By capturing this activity, the researchers could correlate specific brain activity patterns with intended speech. However, a significant challenge arose due to Anns inability to vocalize, leaving no actual audio targets for the AI to match with the brain data during training sessions. To overcome this, the researchers implemented a text-to-speech system to generate simulated audio that the AI could use as a target during training. Cho further elaborates, We also used Anns pre-injury voice, so when we decode the output, it sounds more like her. They even utilized a recording of Ann speaking during her wedding as a source to personalize the synthesized speech, making it resonate more closely with her original vocal characteristics.
To assess the effectiveness of the new system, the research team compared the time taken by the system to generate speech with the initial signals of speech intent emanating from Anns brain. Gopala Anumanchipalli, another researcher involved in the study, noted, We can see relative to that intent signal, within one second, we are getting the first sound out. He also emphasized that the device is capable of continuously decoding speech, allowing Ann to communicate without interruptions. Impressively, this enhanced speed did not compromise the system's accuracy; the new method was shown to decode signals with the same reliability as previous, slower systems.
The decoding process operates in a continuous manner, processing neural signals in small 80-millisecond chunks and synthesizing audio output in real-time. The algorithms developed for decoding the brain signals bear resemblance to those employed by popular smart assistants such as Siri and Alexa. Anumanchipalli commented, Using a similar type of algorithm, we found that we could decode neural data and, for the first time, enable near-synchronous voice streaming. The result is a speech synthesis that is more fluid and natural than ever before.
Another key aspect of this research involved verifying whether the AI model was accurately capturing Ann's intended speech. To evaluate this, Ann was asked to vocalize words beyond the original training data set, including elements like the NATO phonetic alphabet. Anumanchipalli explained, We wanted to see if we could generalize to the unseen words and really decode Anns patterns of speaking. The positive outcome of their findings indicates that the model is successful in learning the foundational elements of sound and voice, demonstrating its potential for broader applications.
While this research remains at the frontier of machine learning and brain-computer interfaces, it represents a significant leap forward in the synergy between these fields. Neural networks show an incredible ability to decode the intricate details of brain activity, paving the way for a future where the gap between humans and computers continues to narrow. The implications of this work could lead to even more advanced communication tools and technologies in the future, transforming the lives of those affected by speech impairments.
In conclusion, the collaboration between UC Berkeley and UC San Francisco marks a pivotal moment in the development of brain-to-speech interfaces, bringing us closer to a world where paralyzed individuals can communicate as seamlessly as anyone else.
Featured image: A researcher connects the brain implant to the supporting hardware of the voice synthesis system. Credit: UC Berkeley